Machine Learning-based Network Intrusion Detection System
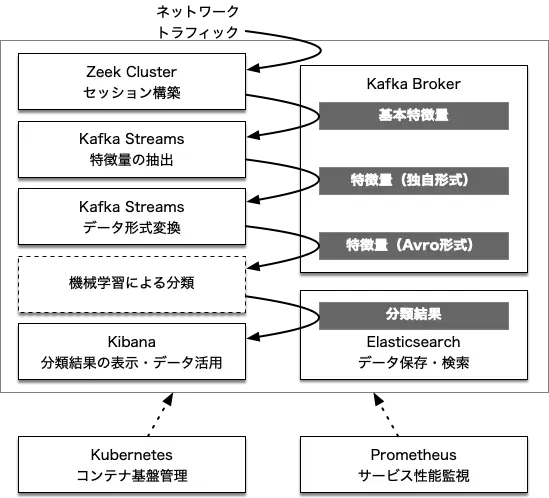
Abstract
Intrusion attacks on servers over the network are increasing every year. Network intrusion detection systems are widely used to detect and block such attacks. However, many systems use pattern files to detect attacks and cannot handle new types of attacks. To solve this problem, research on intrusion detection systems using machine learning is advancing. In this laboratory, we proposed a distributed processing framework called MLNIDS to realize an intrusion detection system using machine learning. We will verify and improve the practicality of this framework from various viewpoints such as detection rate, processing performance, and stability of long-term operation.
Keywords
IDS/IPS, security, network, distributed processing, machine learning